Cubuk E D, Zoph B, Shlens J, et al. RandAugment: Practical data augmentation with no separate search[J]. arXiv preprint arXiv:1909.13719, 2019.
1. Overview
In this paper, it proposes RandAugment
1) Demonstrate the optimal strength of a data augmentation depends on the model size and training set size. (A separate optimization of an augmentation policy on a smaller proxy task may be sub-optimal for learning and transferring augmentation policies)
2) Simplify search space only containing 2 hyperparameters.
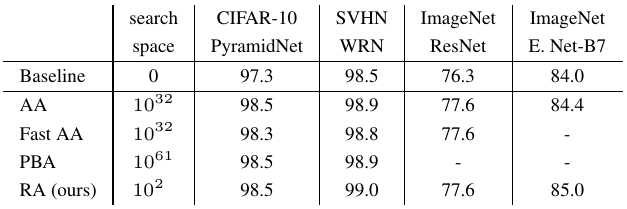
2. Methods
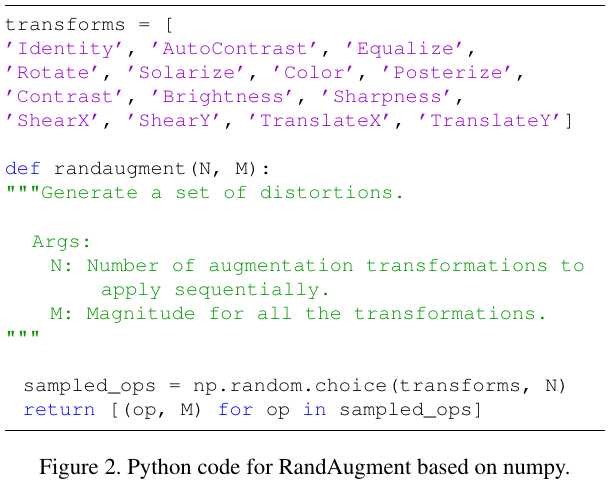
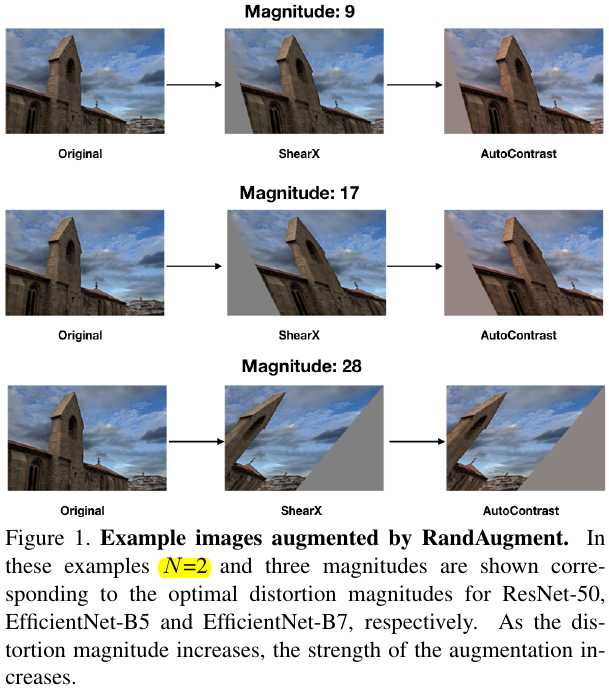
The primary goal of RandAugment is to remove the need for a separate search phase on a proxy task. As a separate search phase significantly complicates training and is computationally expensive.
1) $K=14$. Each transformation with uniform probability $\frac{1}{K}$.
2) RandAugment may thus express $K^N$ potential policies.
3. Experiments
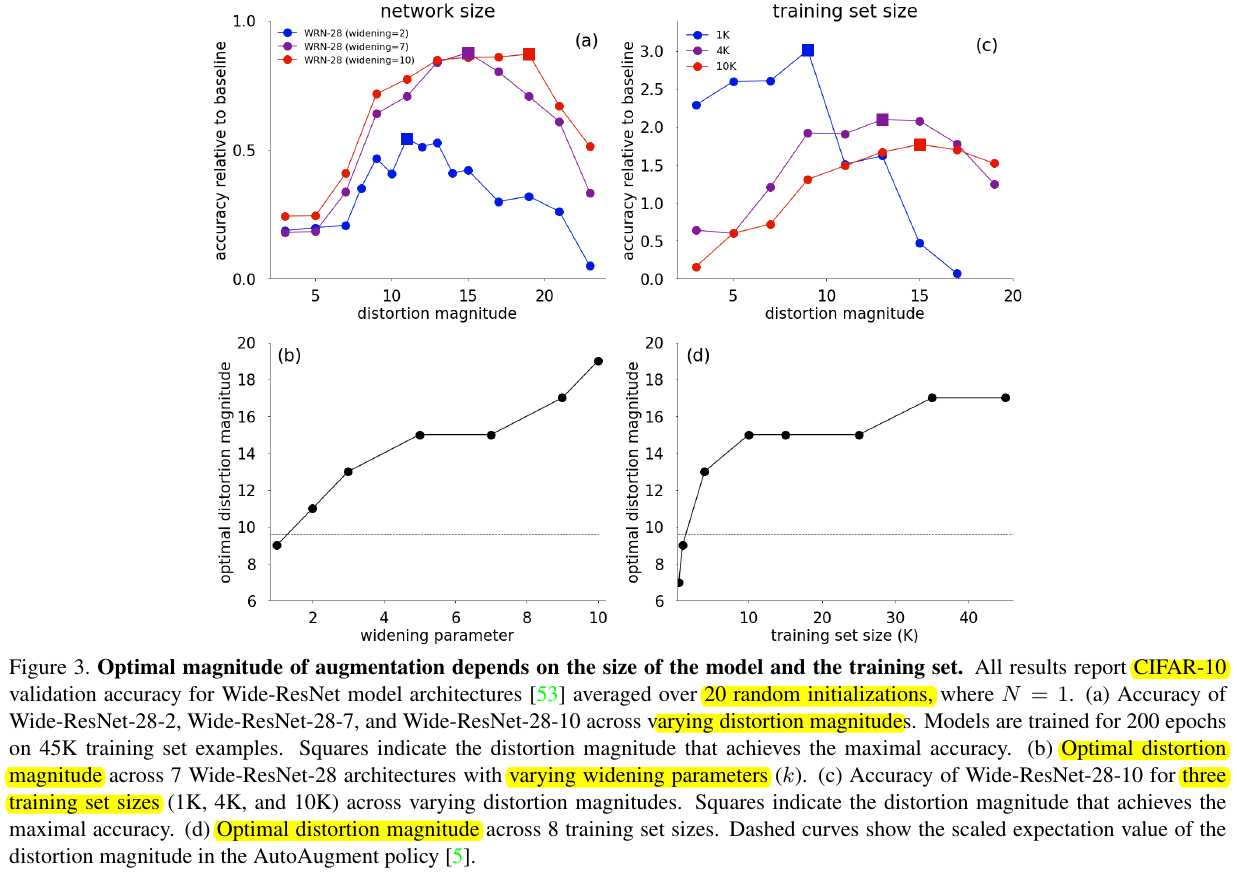